A 2015 Gallup poll showed that 55% of American adults have money invested in the stock market. This is down somewhat from the 2008 high of 65%, but the percentage has not dropped below 52% during the time reported in the survey. This is a significant number of families whose futures are at least partially impacted by long-term market trends. This is because a lot of these investments are money that is tied up in retirement investments, like 401(k)s. That is, by people who put a part of every paycheck into their retirement accounts and won’t touch that money again until perhaps decades later in retirement. For them, what happened to the markets on any given day is completely immaterial. Whether, for example, the Dow Jones Industrial Average shows gains or losses today doesn’t impact their investment strategy, nor will it have any significant impact on their portfolio value when it comes time to begin withdrawing money from their accounts. But how the broad markets change on a long-term scale is hugely important, and completely overlooked by pretty much everybody.
So it makes sense to look at the stock market behavior with a long-term view for a change. That is, let’s not focus on the 52 week average, but the 52 year behavior.
The markets, it turns out, obey three laws. That is, there are three rules governing the behavior of the stock markets, broadly speaking. They may not hold strongly for any particular stock, as one company’s stock price is dependent on a great deal of considerations, but when the marketplace is looked at as a whole, these rules apply.
The three laws governing the long term behavior of the stock markets are:
- Broad market indices grow, on average, exponentially in time.
- An index value at any time will naturally fall within some distribution around its exponential average value curve. This range of values is a fixed percentage of the average value.
- The impact of bubbles and crashes is short term only. After either of these is over, the index returns to the normal range as if the bubble/crash never happened.
Let’s look at each of these in more detail.
First, Law 1: Broad market indices grow, on average, exponentially in time. Below I have plotted the Dow Jones Industrial Average, the Standard and Poor’s 500, and the NASDAQ Composite values from inception of the index to current value. Each of them covers a different span of time because they all started in different years, but they all cover multiple decades. These plots may look slightly different from ones you may be used to seeing since I’m using a semi-log scale. Plotted in this way, an exponential curve will show up as a straight line. Exponential fits to each of the index values are shown in these plots as straight red lines. Each red line isn’t expected to show the actual index value at any time, but rather to show the model value that the actual number should be centered around if it grew exponentially. That is, the red line is an average, or mean, value. If the fit is good, then the red line should split the black line of actual values evenly, which each does quite well.


Some indices hold to the line a bit tighter than others, but this general exponential trend represents the mean value with a great degree of accuracy. This general agreement, which we will see more clearly shortly, is a validation of the first law. The important thing to observe here is that while the short-term changes in the markets are widely considered to be completely unpredictable, the long-term values are not. Long-term values obey the exponential growth law on average, but fluctuate around it on the short-term.
Which brings us to the Law 2: An index value at any time will naturally fall within some distribution around its exponential average value curve. This range of values is a fixed percentage of the average value. If we look at the statistics of the differences between the actual black line values and the red line models, we can understand what the normal range of variation from the model is. That is, the range we should expect to find it in at any time. We can express the difference as a percentage of the model (red line value) for simplicity, and this turns out to be a valuable approach. Consider that a 10 point change is an enormous deal if the index is valued at 100 points, but a much smaller deal if the index is valued at 10,000 points. Using percentages makes the difficulty of using point values directly go away. But it is also valuable because it allows us to observe the second law directly.
The histograms below show how often the actual black line index value is some percentage above or below the red line. These distributions are all centered around zero, indicating a good fit for the red line, as was mentioned previously. And I have colored in green the region that falls within +/- 1 standard deviation from the model value.


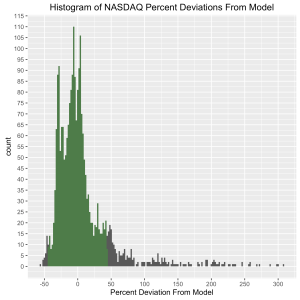
That +/- 1 standard deviation from the model is the rule I used to color in the green region in the charts of index values at the top. If we consider this range to be the range that the index value fall in under “normal” conditions, i.e. not a bubble and not a crash, then we can readily determine what would be over-valued and under-valued conditions for the index. That is, we define and apply a standard, objective, data-based method to determine the market valuation condition as opposed to wild speculation or some other arbitrary or emotional method used by the talking heads.
These over- and under-valued conditions are marked in the (light) red and blue regions on first three plots. Note just how well the region borders indicate turning points in the index value curves. This second law gives us a powerful ability to make objective interpretations of how the markets are performing. It gives us the ability, not to predict day-to-day variations, but the understand the normal behavior of the markets, and how to identify abnormal conditions.
This approach immediately raises two questions: what are these normal ranges for each index, and how often is this methodology representative of the index’s value. Without boring you too much about how those values are calculated, let me simply direct you to the answers in the table below. Note that the magnitude of the effect of tech bubble in the late 1990s and early 2000s on the NASDAQ makes the standard deviation for it larger than the other two. These variations, in the 30-40% range, are large, to be sure. But they take into account how the markets actually behave: how well the companies in the index fit individual traders’ beliefs about what the future will bring.
Index |
1 Standard Deviation (%) |
% Time Representative |
DJIA |
32.5 |
63.4 |
S&P 500 |
30.3 |
70.2 |
NASDAQ |
44.2 |
87.5 |
What can be readily seen here is the psychology of the market. That is, when individual traders start to feel that things might be growing too quickly, approaching overvaluation, they start to sell and take some profits. This leads to a decrease in the value of the index, which then quickly falls back into the “normal” range. The same psychology works in the opposite fashion when the index value shows a bargain. When the value is low, the time is ripe for buying which drives the value back up into the “normal” range. If you look closely at those first three plots, you’ll see how often the index value flirts with the borders of the green region. Actual values might cross this border, but generally not for long. And interestingly, if you calculate this standard deviation percentage for other indices (DAX, FTSE, Shanghai Composite, etc.), you’ll find numbers in the 30s and see precisely the same behavior.
This brings us to Law 3: The impact of bubbles and crashes is short term only. After either of these is over, the index returns to the normal range as if the bubble/crash never happened. The definitions of bubbles and crashes are subjective, and will vary among analysts. But we agree on a few. It is unlikely that anyone will dispute the so-called tech bubble of the late 1990s into the early 2000s. All of the market indices ran far into overvalued territory. Similarly, few would call the crash of 2008 by any other name, with major point drops over a very short period of time. So we will start with these.
An examination of any of the first three plots show precisely this third law in effect for both of these large transitional events. After the tech boom, the Dow, the S&P, and the NASDAQ all fell back to, look to see that this is true, within a few percent of the red line – which is where they would have been had the bubble not happened. The 2008 crash similarly showed a sharp drop down into the undervalued blue zone for all three indices. All three were back into the “normal” range within a year, and have been hovering around the red line for some time now. The three major US indices today are exactly where their long term behavior suggests they should be.
There are plenty of other mini crashes – sharp drops in value due to economic recession. The St. Louis Federal Reserve Economic Database (FRED) lists the dates of official economic recession for the US economy. Overlaying those dates (light red vertical bars) onto the Dow Jones plot shows what happens to the index value during economic recession, and what happens after. This is typically a return to a value close to what it was before.

Overall, even with the crummy performance in the 1970s, the Dow shows over 60 years of average growth that is precisely in line with the exponential model. By the mid 1980s the effect of the languid 70s was gone and the DJIA was right back up to the red line, following the trend it would have taken had the 70s poor performance not happened. In no case, for any of the three indices shown here or foreign indices such as the DAX, the FTSE, the Shanghai Composite, or the HangSeng, has bubble growth increased long term growth, and similarly, never has a crash slowed the overall long term growth.
To wrap up, there is a defining characteristic of exponential growth, one single feature which distinguishes it from all other curves. This defining characteristic is a constant growth rate. It is this constant rate of growth that produces the exponential behavior from a mathematical perspective. Any quantity that has a constant rate of growth (or decay) will follow an exponential curve. Population growth, radioactive decay, and compound interest are all real world examples. And while the growth rate is the most obvious number that can characterize these curves, perhaps the more interesting one is the doubling time (or half life, if you’re thinking about radioactive decay).
The doubling time is the how long it would take for an index’s value to double. The larger the growth rate, the smaller the doubling time. Note that this effect will happen almost 3.5 years sooner for a NASDAQ fund than for a DJIA fund. This, then, is where rubber meets road.
Index |
Mean Annual Growth Rate (%) |
Doubling Time (years) |
DJIA |
6.7 |
10.7 |
S&P 500 |
7.1 |
10.1 |
NASDAQ |
9.9 |
7.3 |
To understand the significance of the market laws in general (the first two, anyway) and these numbers specifically, let’s do an example. Imagine that we receive $1000 in gifts upon graduating from high school. We invest all of that money in an index fund, never adding to it and never withdrawing any money from the fund. We leave it there until we retire at age 65. Assuming we start this experiment at 18, our money, in the DJIA fund, will double 4.4 times over the years (47 years/10.7 year doubling time), giving us about $21,000. Not bad for a $1000 investment. It will double 4.7 times in the S&P 500 fund giving us a slightly higher $25,000 if we went that option. Now consider the NASDAQ, where it will double 6.4 times resulting in almost $87,000.
But that is just the application of the first law, which applies to average growth. We need to consider the second law, because it tells us what range around that mean we should expect. The numbers we need to do this are in the first table above, which express the variability in the value of the index as a percentage of its mean value.
Doing the math, we see that we should expect the DJIA investment at age 65 to be fall roughly between $14,000 and $28,000 (a standard deviation of $6700), the S&P 500 to fall between $17,500 and $32,000 (a standard deviation of $7500), and the NASDAQ to be between $49,000 and $125,000 (a standard deviation of $38,000). Certainly the NASDAQ fund’s variation is quite large, but note that even the low side of the NASDAQ fund’s projected value is still almost double that of the DJIA’s best outcome.
Because some people, like me, process things better visually, here are is the plot. The “normal” range is shaded for each index. Note that by about age 24 the prospect of a loss of principal is essentially gone.

The third law, it should be said, tells us we should expect these numbers to be good in spite of any significant bubbles or crashes that happen in the middle years. Laws are powerful. Use them wisely.